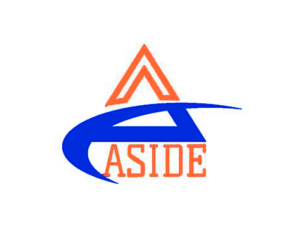
define generative ai 2
Top Generative AI Trends That Will Define 2025 GenAI Trends 2025
What Is Generative AI’s Role In Unlocking Dark Data?
It excels in areas where predefined algorithms can handle well-defined problems, operating with clear, predictable rules that make its decision-making process relatively transparent. At its core, GAI uses algorithms and vast amounts of data to learn patterns. Once trained, it can generate content that mimics what it has learned but with its own unique twist. Where other models have their content reviewed by human trainers in a process called reinforcement learning from human feedback (RLHF), Claude’s was trained with RLHF as well as a second AI model. Reinforcement learning from AI feedback (RLAIF) tasked the “trainer” model with comparing Claude’s behavior against Constitutional AI and correcting it accordingly.
Employees often adopt shadow AI tools to fill gaps in approved technology. Hosting surveys or workshops can uncover the tools they’re using and the reasons behind them. This insight helps pinpoint governance weaknesses and identify opportunities to meet their needs with sanctioned solutions. Treat the policy as a dynamic resource that adapts to new challenges and opportunities, keeping it aligned with the organization’s needs and security priorities. Employees need clear guidance on acceptable AI use, which makes a well-defined Responsible AI policy essential. This policy should outline the types of data that can be processed, prohibited activities, and security protocols everyone must follow.
If, for instance, hallucinating news bots respond to queries about a developing emergency with information that hasn’t been fact-checked, it can quickly spread falsehoods that undermine mitigation efforts. One significant source of hallucination in machine learning algorithms is input bias. If an AI model is trained on a dataset comprising biased or unrepresentative data, it may hallucinate patterns or features that reflect these biases. Retailers, banks and other customer-facing companies can use AI to create personalized customer experiences and marketing campaigns that delight customers, improve sales and prevent churn. Companies can implement AI-powered chatbots and virtual assistants to handle customer inquiries, support tickets and more. These tools use natural language processing (NLP) and generative AI capabilities to understand and respond to customer questions about order status, product details and return policies.
Explainer: What is generative AI? – Science News Explores
Explainer: What is generative AI?.
Posted: Wed, 09 Oct 2024 07:00:00 GMT [source]
BERT is designed to understand bidirectional relationships between words in a sentence and is primarily used for task classification, question answering and named entity recognition. GPT, on the other hand, is a unidirectional transformer-based model primarily used for text generation tasks such as language translation, summarization, and content creation. If the three-part logical structure of an AI sounds familiar, that’s because neural nets have the same three logical pillars. In fact, from IBM’s perspective, the relationship between machine learning, deep learning, neural networks and artificial intelligence is a hierarchy of evolution. It’s just like the relationship between Charmander, Charmeleon and Charizard.
Large language models
Picture AI crafting artwork, composing melodies, or designing fashion pieces tailored to the latest trends. It can transform text descriptions into images, helping artists bring their concepts to life in no time. Reinforcement learning (RL) changes things up by teaching AI through trial and error. The AI acts like an agent exploring an environment, making decisions to achieve rewards. So, while traditional AI is a whiz at analyzing data and handling repetitive tasks, generative AI is where the magic happens, bringing new media to life. Artificial intelligence includes a range of technologies, with “traditional” AI and generative AI (GAI) at the forefront.
Organizations feeding internal data into GPT might expose themselves to cybersecurity breaches or violate data protection regulations. For industries such as fashion, AI can generate original designs or assist in refining patterns based on trends, making it easier for brands to innovate quickly. In media, AI-generated content personalization is increasingly used to engage diverse audiences by curating experiences based on user interests.
Integrate mechanisms for human oversight in critical decision-making processes. Define clear lines of accountability to ensure responsible parties are identified and can be held responsible for the outcomes of AI systems. Establish ongoing monitoring of AI systems to identify and address ethical concerns, biases or issues that may arise over time. Ensure that the training data used to build AI models is diverse and representative of the population it is meant to serve.
You can opt out of it using your data for model training by clicking on the question mark in the bottom left-hand corner, Settings, and turning off “Improve the model for everyone.” There are also privacy concerns regarding generative AI companies using your data to fine-tune their models further, which has become a common practice. For example, chatbots can write an entire essay in seconds, raising concerns about students cheating and not learning how to write properly.
Limitations of GPT-4o
Implementing responsible AI practices at the enterprise level involves a holistic, end-to-end approach that addresses various stages of AI development and deployment. Apply techniques like re-sampling, re-weighting and adversarial training to mitigate biases in the model’s predictions. Practitioners need to be able to understand how and why AI derives conclusions. For more information, see how generative AI can be used to maximize experiences, decision-making and business value, and how IBM Consulting brings a valuable and responsible approach to AI.
Since Conversational AIis dependent on collecting data to answer user queries, it is also vulnerable to privacy and security breaches. Developing conversational AI apps with high privacy and security standards and monitoring systems will help to build trust among end users, ultimately increasing chatbot usage over time. To understand the entities that surround specific user intents, you can use the same information that was collected from tools or supporting teams to develop goals or intents.
Hardware failures can cascade, like the Great Northeast Blackout of summer 2003, or when Texas froze solid in 2021. We also live in a timeline where a faulty firmware update can brick your shoes. In practice, the main differences between the two are latency and horsepower. Local processing is always going to be faster than a data pipeline beholden to ping times.
In May 2024, OpenAI announced the multilingual and multimodal GPT-4o1, capable of processing audio, visual and text inputs in real time. Accuracy is a key component of how successful the use of AI is in everyday operation. By running simulations and comparing AI output to the results in the training data set, the prediction accuracy can be determined.
Instead, they can query, calculate and generate reports through natural language. A business can take a foundation model, train it on its own data, and fine-tune it to a specific task or a set of domain-specific tasks. GPT-4 and other foundation models are trained on a broad corpus of unlabeled data and can be adapted to many tasks. Many generative AI art models are trained on billions of images from the internet. This content often includes artwork and images produced by artists and creatives. These images are then reimagined and repurposed by AI to generate your image.
Diverse teams can bring different perspectives to the table, helping to identify and rectify biases that may be overlooked by homogeneous teams. Let’s look at the properties that make up the “Pillars of Trust.” Taken together, these properties answer the question, “What would it take to trust the output of an AI model? ” Trusted AI is a strategic and ethical imperative at IBM, but these pillars can be used by any enterprise to guide their efforts in AI. Oh, okay, there This is why we lampoon the IoT with loving names like the Internet of Shitty Things and the Internet of Stings. (Internet of Stings devices communicate over TCBee-IP.) But the AIoT isn’t something anyone can sell.
This is a way of churning through the heap of example data in search of patterns. Things that often show up together in the data get placed close together in these maps. IBM® Granite™ is our family of open, performant and trusted AI models, tailored for business and optimized to scale your AI applications. In customer support, chatbots and virtual agents are stepping up their game. These AI-driven tools can handle everything from resetting passwords to processing orders in a flash.
How generative AI is used in business intelligence
To do so, enterprises must have an infrastructure that properly manages data and supports AI technology. Having a strong data governance framework helps keep data available to all relevant stakeholders and secure from data breaches. While the advancement in Generative AI suggests that machines will be able to take over the world, let’s remember how machines generate their output. In large language models, when given a prompt, machines grab the most probable next word, line of code, or image. But the creativity occurred when humans put information into the dataset. A machine doesn’t understand what it means to have a broken heart but will finish the sentence “When he left me, he broke my…” with “heart” because millions of people have written that.
Applications for AI in content marketing include automated content creation. Through these processes, AI tools generate high-quality blog posts, social media updates and ad copy based on specific keywords, topics and styles. Other researchers have developed techniques to help explain how models arrive at specific conclusions.
Generative AI vs. predictive AI: What’s the difference?
Generative AI is, therefore, a machine-learning framework, but all machine-learning frameworks are not generative AI. There are numerous ways AI can positively impact the practice of medicine, whether it’s through speeding up the pace of research or helping clinicians make better decisions. There have been methods statisticians and researchers use to study qualitative, unstructured information for many decades.
- Generative BI tools work the same way other generative AI-powered tools do.
- At the time of its release, GPT-4o was the most capable of all the OpenAI models in terms of both functionality and performance.
- “Agentic” AI — where teams of generative AI “agents” work together to solve multi-step, multivariable problems — is often cited as the future of the technology.
- This training process is compute-intensive, time-consuming and expensive.
Most generative AI models lackexplainability, as it’s often difficult or impossible to understand the decision-making processes behind their results. Conversely, predictive AI estimates are more explainable because they’re grounded on numbers and statistics. But interpreting these estimates still depends on human judgment, and an incorrect interpretation might lead to a wrong course of action.
The most obvious method is to invest directly in businesses that run generative AI services, such as Adobe (ADBE -0.43%) or Microsoft (MSFT -0.59%). The hardware that makes it possible is another simple option, including AI accelerator specialist Nvidia (NVDA -3.12%) and computer memory maker Micron Technology (MU -1.57%). You have probably heard the term “generative AI” tossed around in tech circles or investment discussions. It’s one of those buzzwords that seems to be on everyone’s lips nowadays, starting when OpenAI released the ChatGPT tool in November 2022.
Regularly assess models for fairness, especially regarding sensitive attributes such as race, gender or socioeconomic status. Provide clear documentation about data sources, algorithms, and decision processes. Users and stakeholders should be able to understand how AI systems make decisions.
How do transformer models work?
This creates continuity within the customer experience, and it allows valuable human resources to be available for more complex queries. Overall, conversational AI apps have been able to replicate human conversational experiences well, leading to higher rates of customer satisfaction. Frequently asked questions are the foundation of the conversational AI development process. They help you define the main needs and concerns of your end users, which will, in turn, alleviate some of the call volume for your support team.
Human cognitive intelligence characterizes how we summarize, abstract and synthesize stories about our experience of interacting in the physical world and with other humans, animals and machines. The stories we compose summarizing our understanding of these things are what cognitive AI processes. On the creative front, GAI is changing how we approach content generation. Tools like ChatGPT for text and Dall-E or Canva for images allow users to produce original, high-quality work in no time. Media, marketing, and design professionals benefit from this, as it lets them create tailored content faster and offer more engaging, personalized experiences.
In another vein, researchers demonstrated LLMs’ capacity to categorize qualitative data. This process, known as coding, traditionally requires human evaluators to assign labels to text. Manual coding is time-consuming, often necessitating data sampling rather than comprehensive analysis.
A transformer model is a type of deep learning model that was introduced in 2017. These models have quickly become fundamental in natural language processing (NLP), and have been applied to a wide range of tasks in machine learning and artificial intelligence. LLMs allow users to leverage natural language to interact with sets of unstructured data and generate summaries and insights in real time.
What Is Artificial Intelligence (AI)? – ibm.com
What Is Artificial Intelligence (AI)?.
Posted: Fri, 09 Aug 2024 07:00:00 GMT [source]
Learn an agile AI approach that enables organizations to innovate quickly and reduce the risk of failure. We surveyed 2,000 organizations about their AI initiatives to discover what’s working, what’s not and how you can get ahead. Learn how your organization can harness the power of AI-driven solutions at scale to reinvent and transform your business in ways that truly move the needle. Learn how to select the most suitable AI foundation model for your use case. Learn about the new challenges of generative AI, the need for governing AI and ML models and steps to build a trusted, transparent and explainable AI framework. Spelling out how you will use the AI model—as well as any limitations on the use of the model—will help reduce hallucinations.
Generative AI can be used at any stage of the business intelligence process, but it is most commonly used to support data collection, data analysis, data visualization and action planning. While general-purpose gen AI models can perform many BI functions, many organizations opt for the more specialized BI tools and models instead. These typically grant organizations more control over how their data is used. In this way, generative BI enables self-service analytics for users across the organization, regardless of skill set.